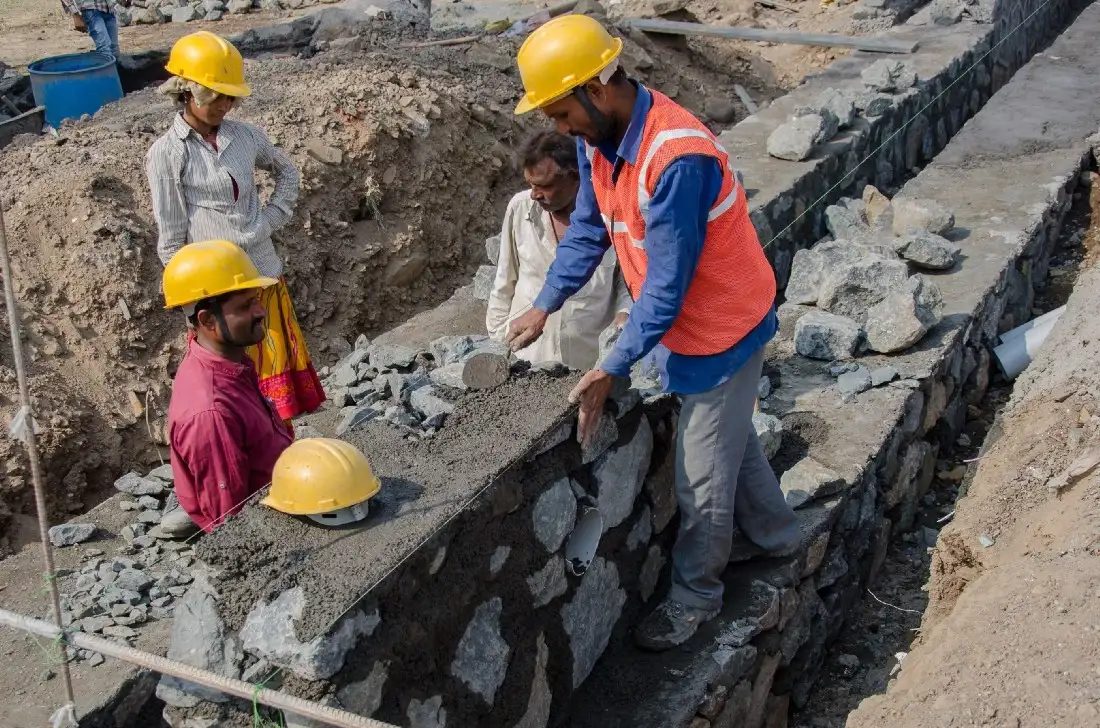
In the last few months, we’ve arrived at a theoretical model of a potential community platform with data cooperatives through literature review and conversations with industry leaders from SEWA, Platform Coop, ICAAP and a multitude of knowledge level gig workers. As a logical next step, we have started talking to gig workers across the skill spectrum and geographies. Currently, this involves focusing on construction workers from Mumbai and driving and delivery professionals in Bangalore. We spoke to a total of 200 out of our target for 4000 individuals over the course of the past two weeks. While we will be spending the next few months scaling up the source and nature of data collected, these are the early insights we have been observing from our on-going surveys in different parts of the country.
Community owned banking may be the future
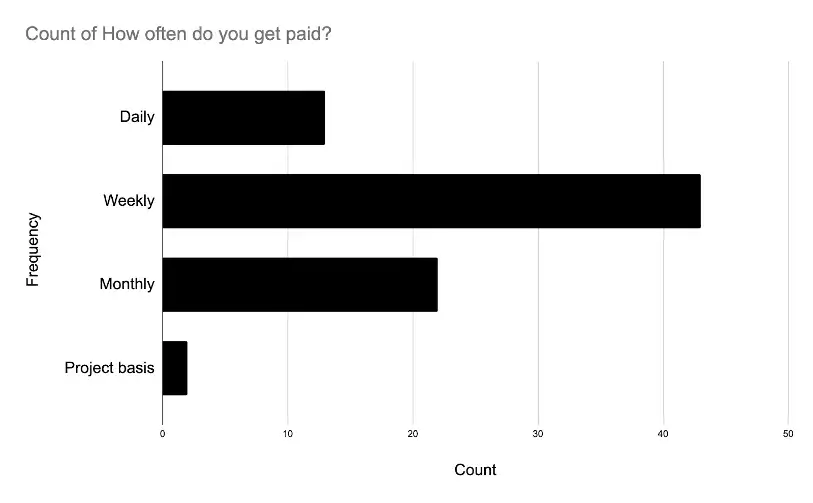
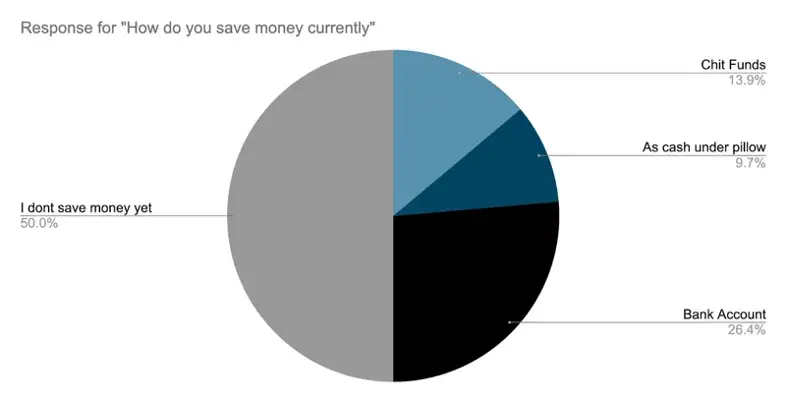
A high number of individuals report not earning sufficiently. ~14% of the users mentioned using chit-funds. For the uninitiated, a chit fund is a type of rotating savings and credit association system practiced in India[1]. Chit fund schemes may be organised by financial institutions, or informally among friends, relatives, or neighbours. In some variations of chit funds, the savings are for a specific purpose. Chit funds are often microfinance organisations. We see this as a proxy for community based banking. This resonates with how SEWA bank was born. Since the poor were unbankable by large (nationalised and private) banking institutions, a group of self employed women came together to start a bank under a co-operative model. Everything about the bank is determined by SEWA members — the bankers and board members are organization members, the loan rates are set by members, the funds are entirely from members [2]. The NFC (Near Field Communication) based card that we had proposed in the previous blog post relies on existing behavior among the target group and focuses on digitising their interactions. The table below shows the amount of money and number of members that are already being serviced by SEWA.
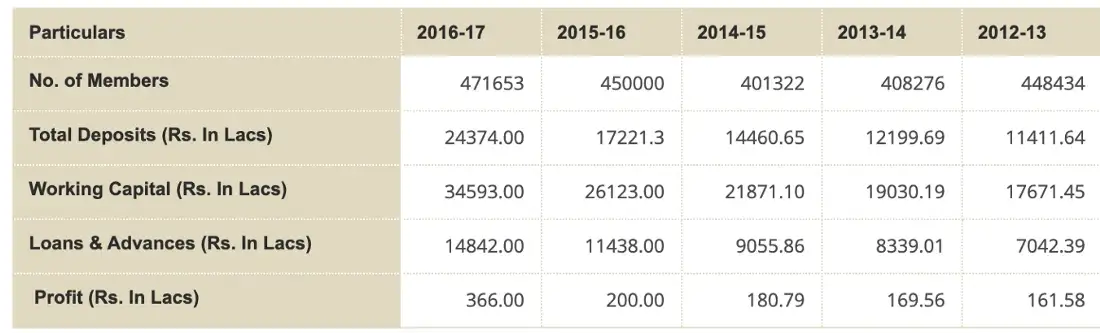
Borrowing Behavior Among Gig Workers
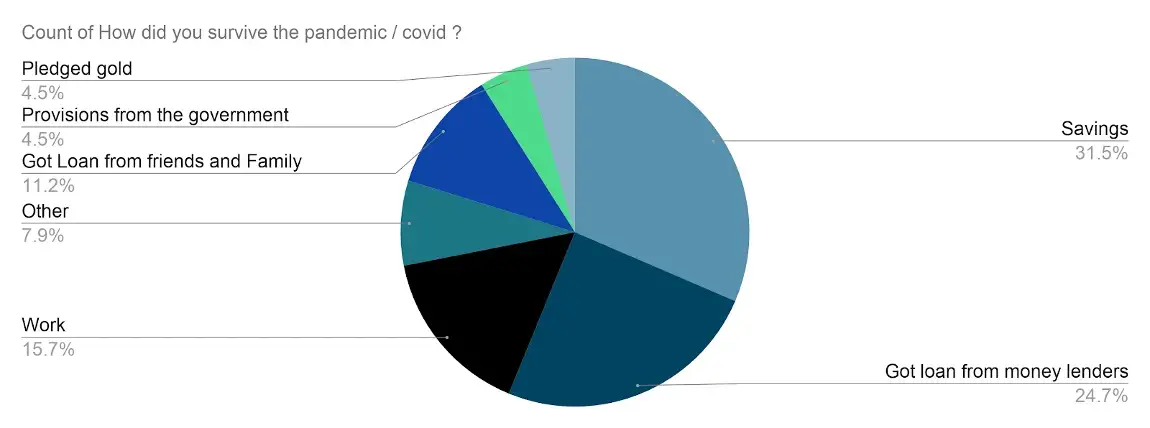
We see two key behavioural patterns here. One, that most of the individuals we speak to have no forms of credit — formal or otherwise. In the cases where debt does exist it is typically for a personal emergency. In those instances, individuals typically rely on existing savings. In the absence of which a money lender is approached instead of formal banking outlets. The role a community plays is evident here too, as we see that over 11% of individuals rely on friends and family for immediate loan.
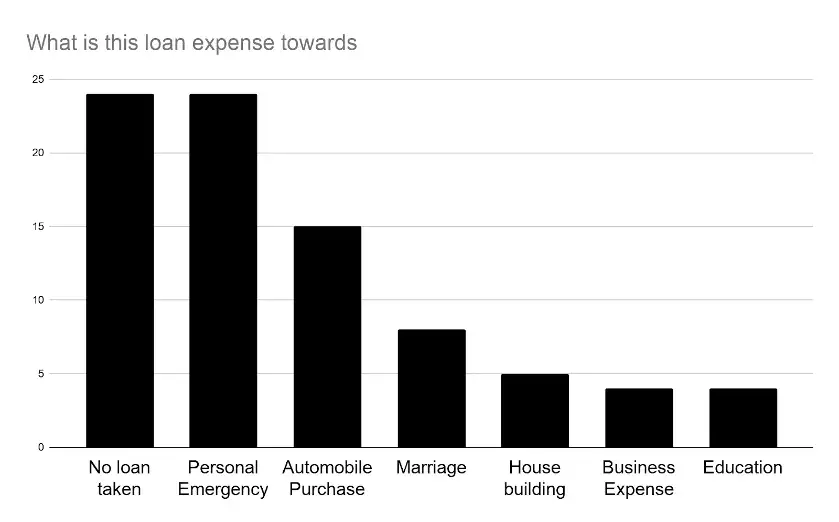
As shown in the graph above, a common alternative use for loan is the purchase of automobiles. In these instances, the individual knows that a clear return on investment can be made as the opportunities for employment rises once a delivery boy has access to a bike. This data however may be skewed because of the fact that the majority of our survey respondents were delivery personnel. The preferences may drastically change as we expand to individuals from other backgrounds and skill-sets.
The Need For Data Portability and Interoperability
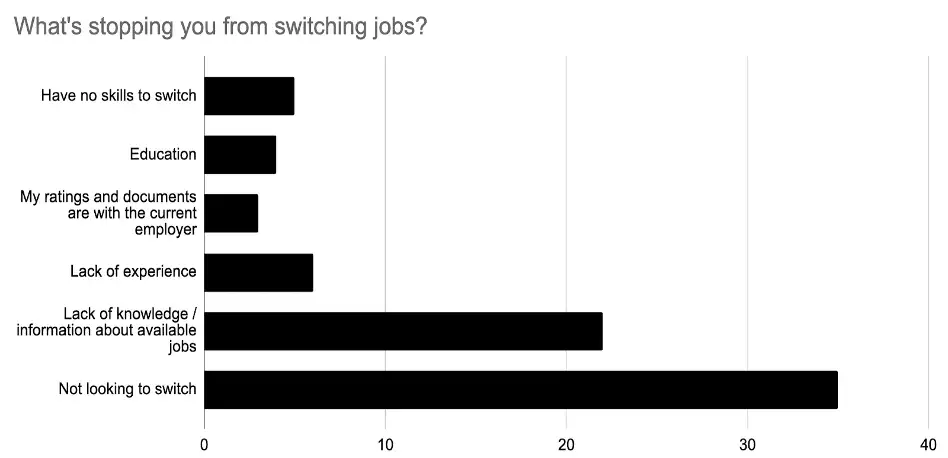
We noticed that even in the absence of conversations around data-ownership and its portability, survey respondents have shown a sensitivity towards the lack of means to port their data. This presents a case for data ownership but needs to be explored deeper cognizant of the framing bias inherent to primary research. Although existing identity layers like Aadhar make it possible for identity to be verified in a fraction of the time it used to take, little work has been done in relation to enabling individuals to port their skills data. Information such as frequency of rides, ratings from passengers and reviews for deliveries done could fetch a premium on the salary if the gig worker performed exceptionally well. Similarly, employee related red-flags could also be spotted by a potential employer when individuals switch jobs. Portability of performance-related information and skills data therefore has a direct impact on the financial well-being of the individual. We need to explore more means to make it more portable between existing organisations.
Communities Critical for Migration & Job Hunting
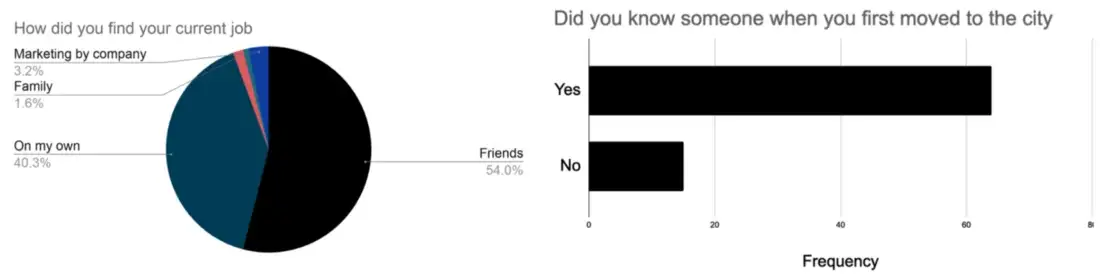
Migration in the target-group we spoke to is heavily reliant on knowing an individual in the city where a job is available. The key reason for individuals to switch their places of dwelling has often been the presence of a family member. We also notice that individuals tend to bring their immediate family towards the city as soon as it is possible. In terms of finding a job opportunity, knowing an individual with access to information related to the job has been the most critical.
What’s next? These are some of the early observations we have made on the basis of speaking to close to 200 individuals over the course of past two weeks. Our immediate focus is to complete the collection of data-sets and expand on speaking to industry experts about how data ownership in a community environment can contribute to the overall well-being of an individual. We have already observed that taking elements of platform coops like SEWA into our existing model can help us improve our model. Please note that the model may change as we speak to more gig workers and people working directly with gig workers.
References:
Modelling credit and savings behaviour of chit fund participants — Preethi Rao (GatesOpenResearch)
SEWA coop Bank — Rekha Datta (Spring 2003). “From Development to Empowerment: The Self-Employed Women’s Association in India”. International Journal of Politics, Culture and Society. 16 (3): 351–368. JSTOR 20020171.